Dash Chrisner (Arch ‘18), Mary Dwyer(mechE ‘19), Eva Gabrielson (Art ‘19), Nicole Lindner (Art ‘20) worked with the Coalition for the Homeless, to analyze a dataset on an eviction prevention program. This program provides people who are on the verge of eviction with a one time grant of up to $1000.
The student team examined two things related to this dataset. First, they tried to build a predictive model and determine if there were any major predictors such as age, race, gender, etc… that influenced whether or not someone would receive the grant. They found that the biggest predictors were the amount of money owed, and the neighborhood the person at risk of eviction lived in. The fact that no other demographic information had a large influence on the prediction indicated that there appears to be little to no bias in the Coalition for the Homeless selection of grant awardees.
The other aspect examined was to overlay the locations of grant awardees with a dataset of bad landlords, as provided by the NYC public advocate. By comparign these locations, there are some obvious areas of overlap as would be expected. Neighborhoods with many ‘bad landlords’ in general seem to have a higher amount of people at risk of eviction. However, there were some neighborhoods, with a high amount of ‘bad landlords’, that currently do not have a high amount of evictions. Further investigation will study what is different about these neighborhoods, and perhaps an intervention strategy can be developed to prevent future evictions in those areas.
See the gallery below for some of their visualizations, and pdf versions of the maps are here and here. To read more details, have a look at their final report
Gallery
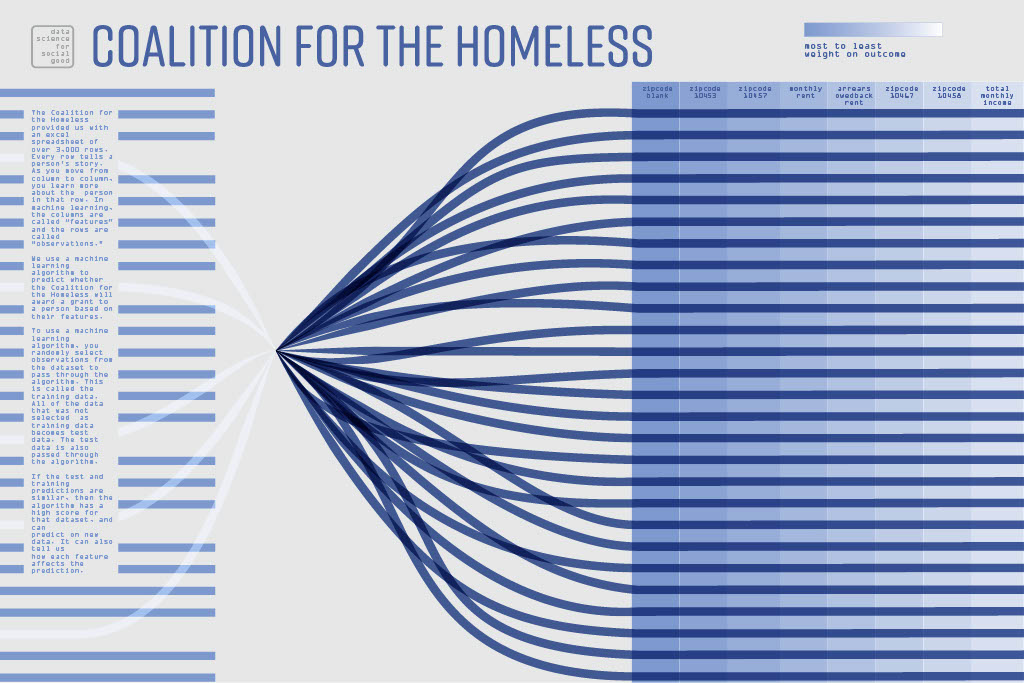
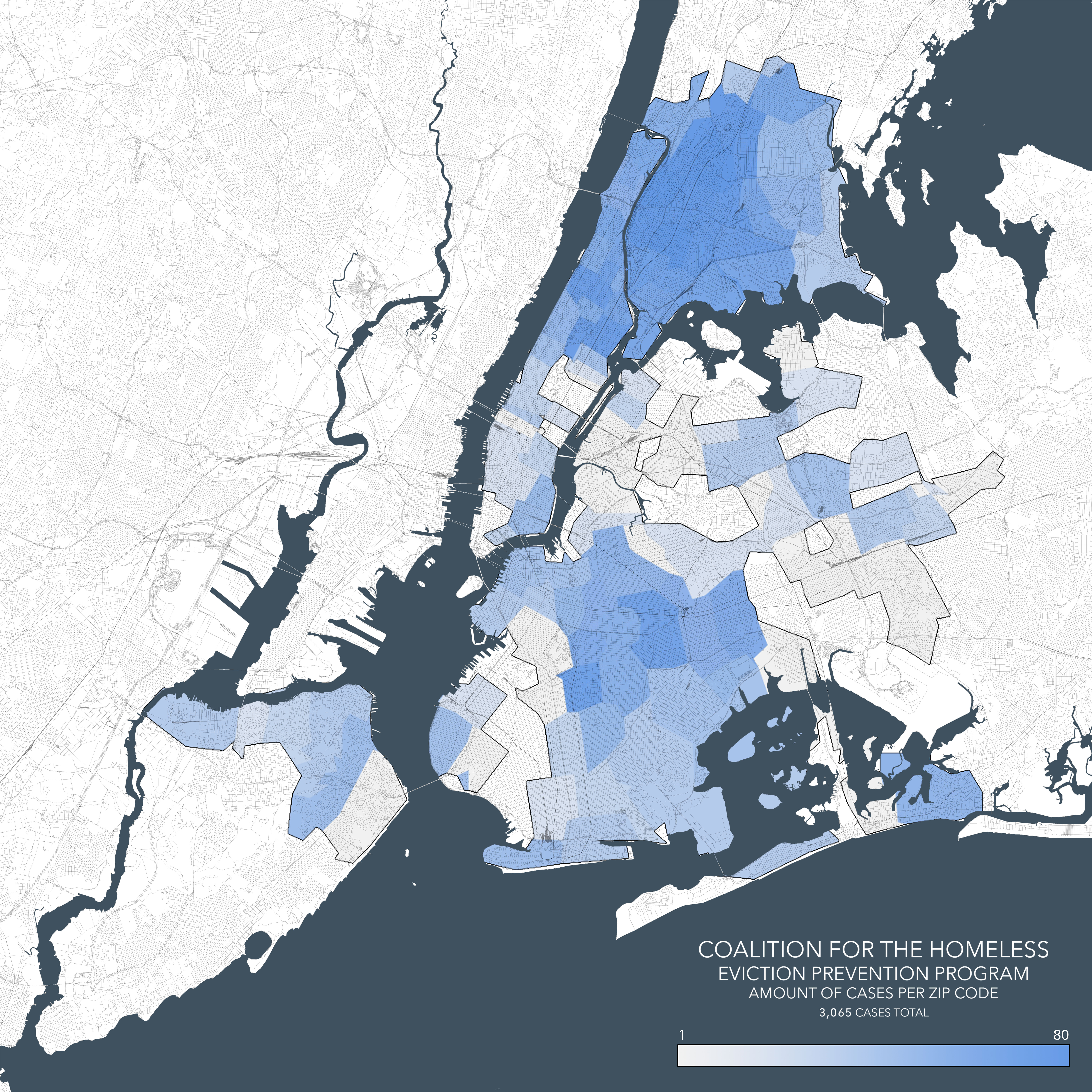
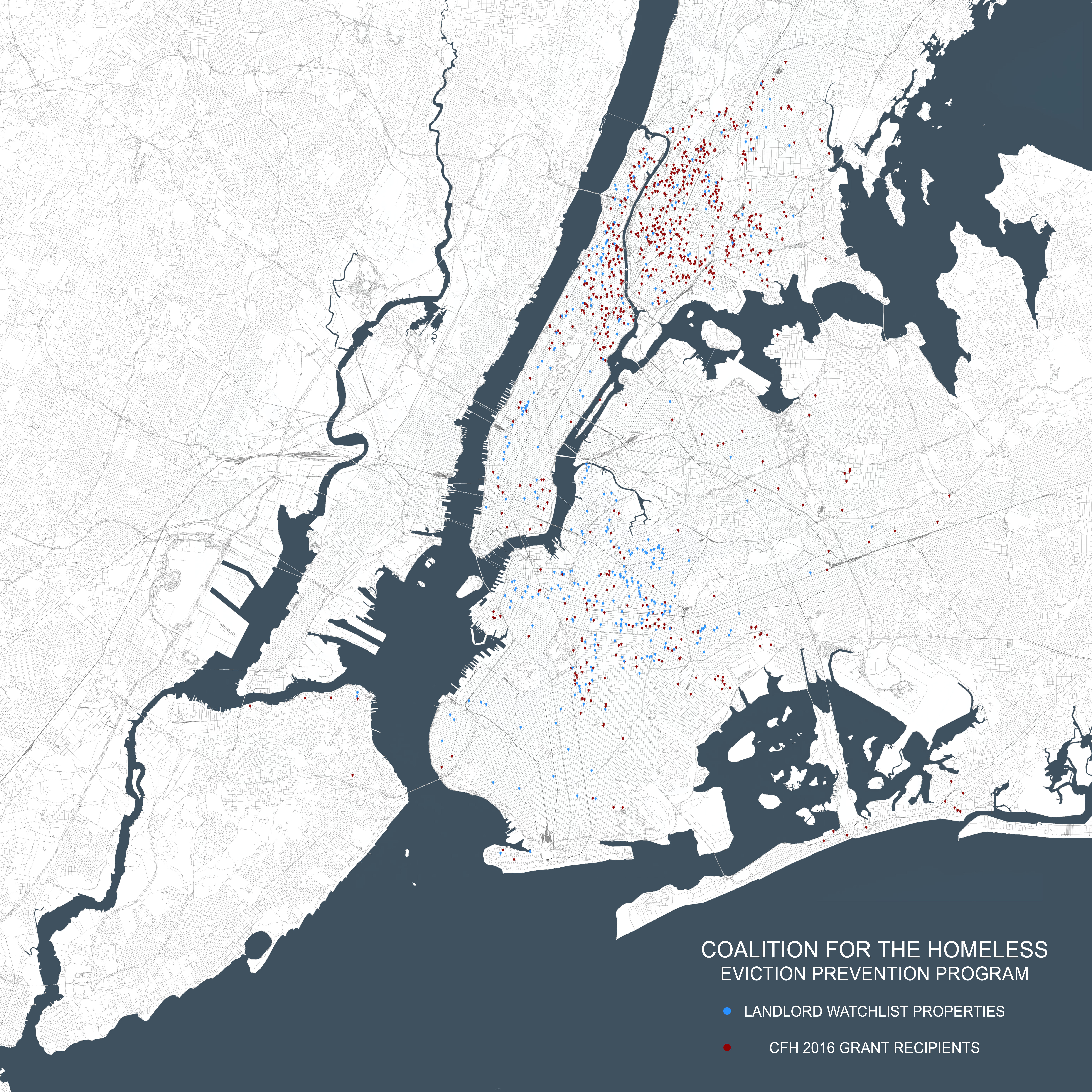